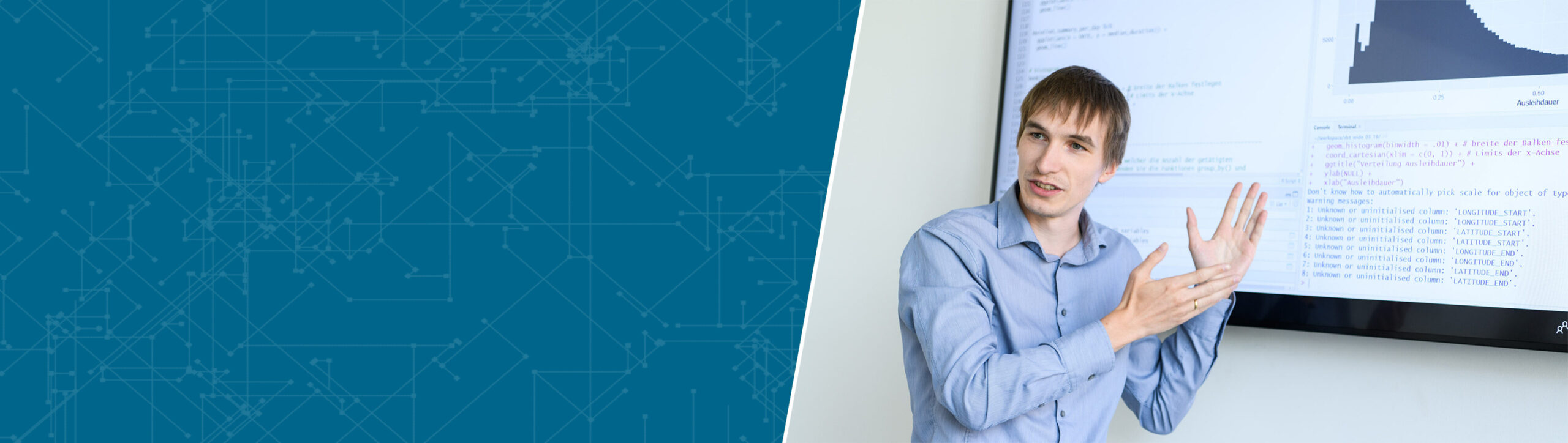
R training in Data Science
In our leading R training courses, we teach you the diverse possibilities of the free programming language in a practical and comprehensive manner.
Our training courses range from beginner courses to courses for advanced R users and from data management to visualization of results – remotely or at your site. Upon request, we are also happy to customize the training content according to your individual needs.
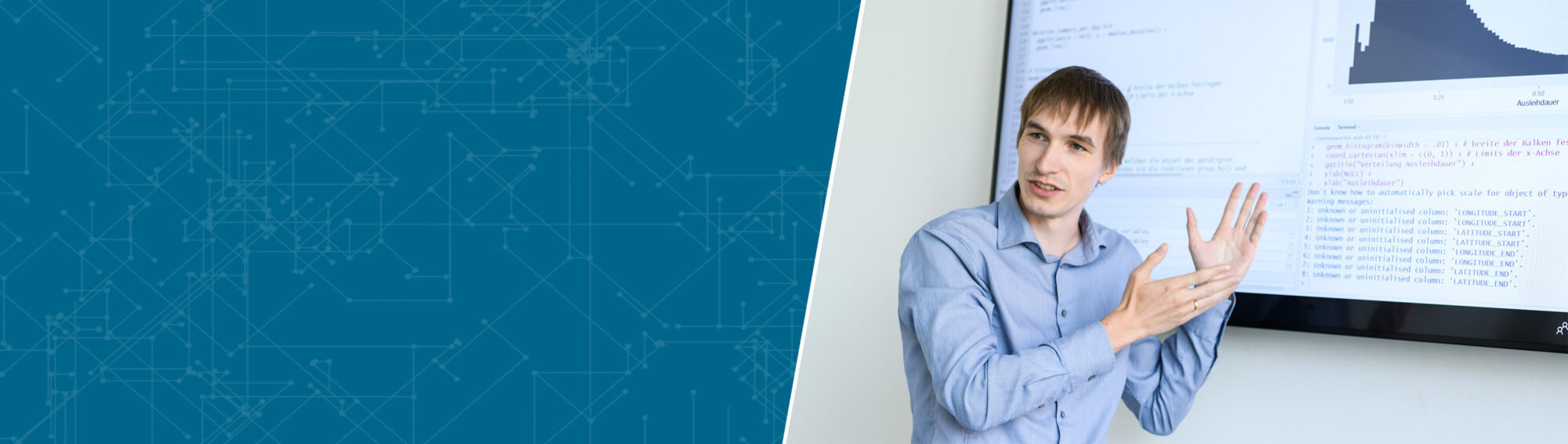
R training in Data Science
In our leading R training courses, we teach you the diverse possibilities of the free programming language in a practical and comprehensive manner.
Our training courses range from beginner courses to courses for advanced R users and from data management to visualization of results – remotely or at your site. Upon request, we are also happy to customize the training content according to your individual needs.
Our R trainings
R is one of the leading programming languages for Data Science. Our R training courses for companies, universities and graduate centers are regularly evaluated and rated as very good. Over 1,500 satisfied participants speak for the quality of our R trainings.
Become an R expert with us.
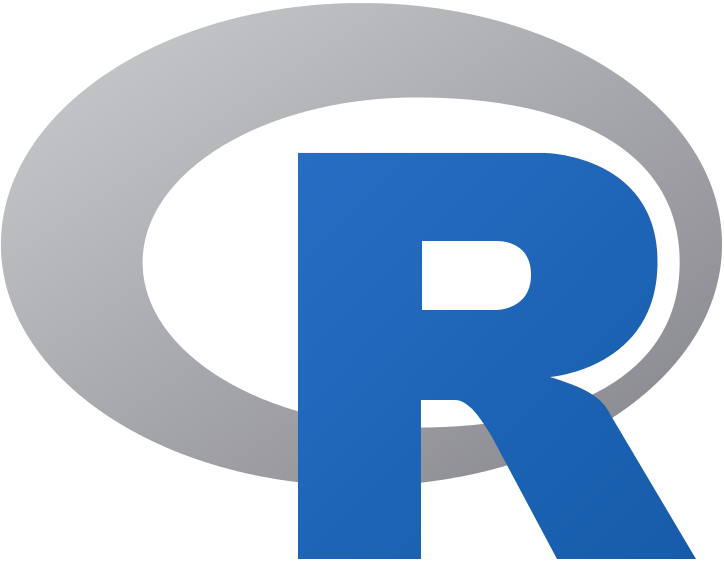
Our two most popular R courses
Introduction to R
The open source language R is one of the best solutions for data analysis, data visualization, data mining and predictive analytics. With R, a unique standard of functionality, quality and actuality can be achieved.
The course is intended as an introduction to R and its basic functionalities. It makes it easier for participants to get started with R by getting practical tips and exercises. This basic course serves R beginners without previous knowledge as a starting point in order to use R in further individual application scenarios.
The objective of the course is to teach the participants the logic and terminology of R and to lay the foundation for working with R independently.
Course content:
- First steps into R
- Concept and philosophy of R
- Data structures and their properties
- Importing data
- Data management
- Data analysis with R
- For loops and control elements
- Visualizations with R
- Introduction to the leading IDE RStudio
—
Recommended course length: Two days
Machine learning with R
Use machine learning and data mining algorithms in R to develop artificial intelligence applications based on your data.
A selection of the training topics:
- Introduction to the basic terms of machine learning
- Introduction to machine learning algorithms such as decision trees, random forest, gradient boosting machine
- Introduction to a methodical approach in the development of machine learning models
- Typical steps in data preparation such as feature selection or data transformation
- Creation of training and test data sets
- Introduction of validation techniques such as cross-validation or bootstrapping
- Introduction and interpretation of different metrics for measuring success such as:
- For classifications: Accuracy, sensitivity, specificity
- For regression: RMSE, MAE, MAPE, …
- Interpretation of ROC curves
- Tuning of parameters
- Introduction of common machine learning packages
—
Target group: This course is aimed at participants with initial programming experience in R.
Recommended course length: Two days
Shiny-Applications with R
As a Full Service Certified Partner of Posit and leading Shiny expert, we enable you to independently develop successful Shiny applications for productive use.
Topic Overview
- Introduction to Shiny
- Philosophy of Shiny: reactive programming
- Data structures and their properties
- User Interface Design
- Workflow for developing a Shiny application
- Extension packages around Shiny
- Do’s and Don’ts for the productive use of Shiny applications
—
Target group: This course is aimed at participants with initial programming experience in R.
Recommended course length: Two days
Customized individual training
You already know the basics of Data Science with R and would like to deepen your knowledge? We would be happy to create an offer for you for an individual training – tailored to your desired topics.
A selection of additional training courses
Date and time
We offer our R-contents as a individual training at a suitable date of your choice.
Location
We offer you the possibility to conduct your training at your site or remote.
Price
Please contact us, we will be pleased to submit you an individual offer for your training.
A selection of our references
What our clients say
“The R training courses from eoda have been very helpful for all of us. We found the training courses extremely successful, challenging and adapted to the learning pace of the group at all times. “
Thomas Feher | Departmental Officer Corporate Controlling | ARAG SE
“The content, structure, pace and presentation of our Data Science training from eoda were very good – even in remote format. Questions and spontaneous requests of the participants were well addressed. The training content will be very helpful for our upcoming projects. We will be very happy to come back to eoda if we need a Data Science training again.”
B. Fischer | Aktuary | Finance & Corporate Management | Süddeutsche Krankenversicherung a.G.
„The feedback from colleagues on eoda’s introductory R course was entirely positive. Above all, the practical exercises were very helpful in order to be able to try out the knowledge imparted directly themselves.“
Sinikka Junginger | Department Marketing Insights | LUSINI Solutions GmbH
Discover also our Data Science Coaching – for your very individual Deep Dive into Python and the success of your data projects.
From data management to visualization and from statistical methods to deep learning: With our Data Science Coaching we expand our empowerment offer for you and we are your guide in the huge Data Science cosmos. Our coaching stands for very personal support and the direct application of the learned knowledge in your concrete application area. Through the feedback and tips and tricks of our coaches, you not only ensure your learning success, but above all your project success.
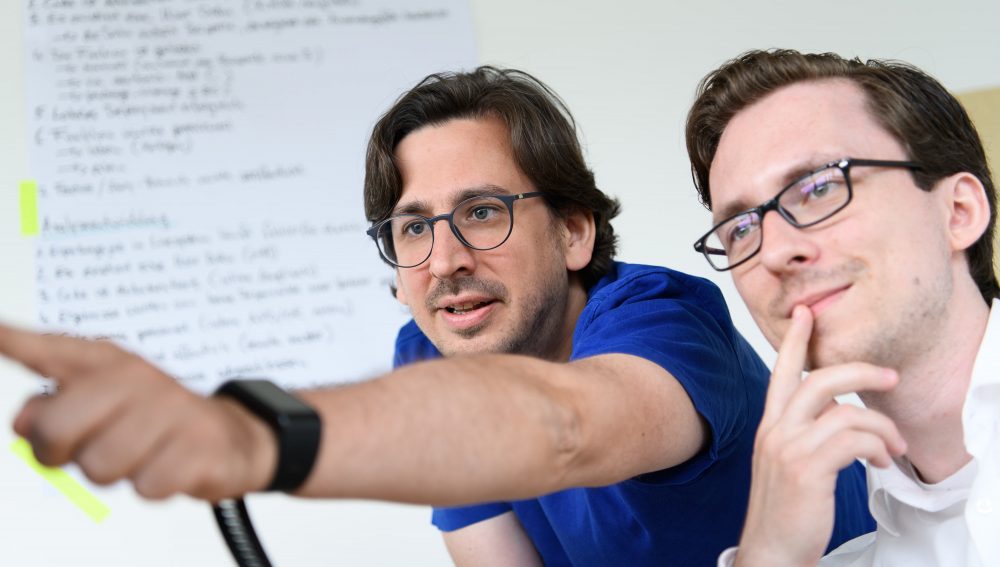
Get started now – we will guide you through the process!!